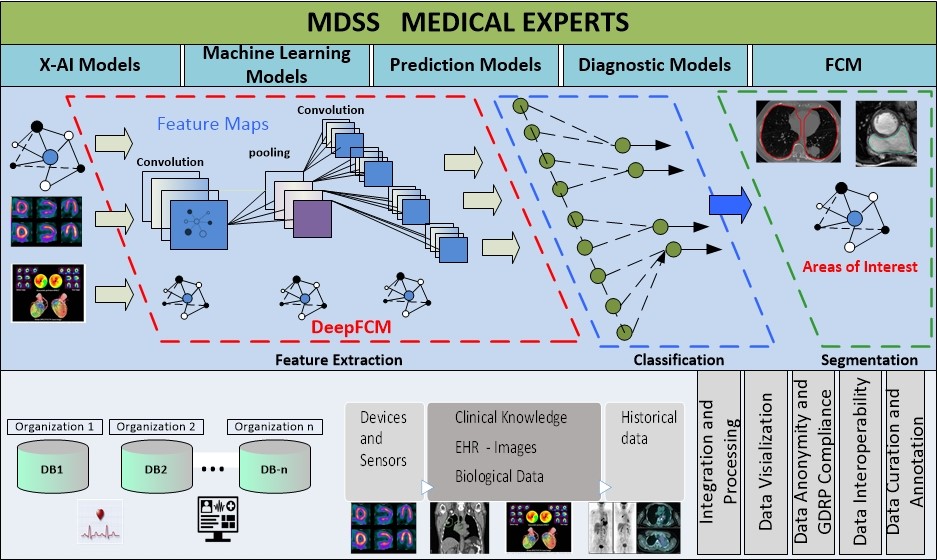
Rationale, the problem and the need
Recently Artificial Intelligence (AI) has significantly supported healthcare intervention decisions via customized Medical Decision Support Systems (MDSS). A repertoire of predictive models based on boosted trees, random forests and straightforward Deep Learning (DL) have provided highly accurate predictions for multi-parameter and complex designs in medicine. However, these systems are limited since, contemporary Machine Learning (ML) models are unable to interpret and explain a physician’s thoughts and actions in patient treatments. There is a need to introduce a model-driven XAI and contextually relevant MDSS that will utilize: (a) the dynamic capabilities of FCMs for modeling complexity, (b) the advances in Deep Learning and XAI, and (c) the “Cause and Effect Reasoning” fuzzy model of human cognitive skills to inherently integrate the notion of interpretability and explainability in medical professional decision making.
The EMERALD breakthrough vision
EMERALD takes a unique, holistic approach to patient-specific predictive modeling and MDSS development by extracting and integrating knowledge from new research, clinical tests and EHR using advanced analytic techniques. ICT technologies (such as Data Mining, Deep Learning (DL) and Advanced Fuzzy Cognitive Tools) will play a key role in EMERALD enabling the analysis simplification of large patient data collections, explainability of decisions made and thus allowing the development of personalized predictive MDSSs
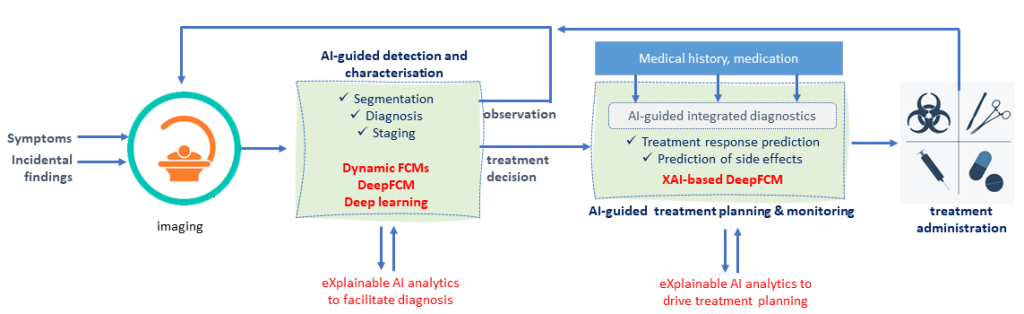